27 Mar 2024
On March 14, the internationally renowned academic journal Nature released a special report titled “How AI is being used to accelerate clinical trials” in the Nature Index Health Science supplement. The article explores the applications, achievements, and challenges of AI in clinical trials, supported by case studies from multiple countries worldwide. Taimei Technology’s AI applications in data collection and mining were specifically cited as examples of innovation in the field.
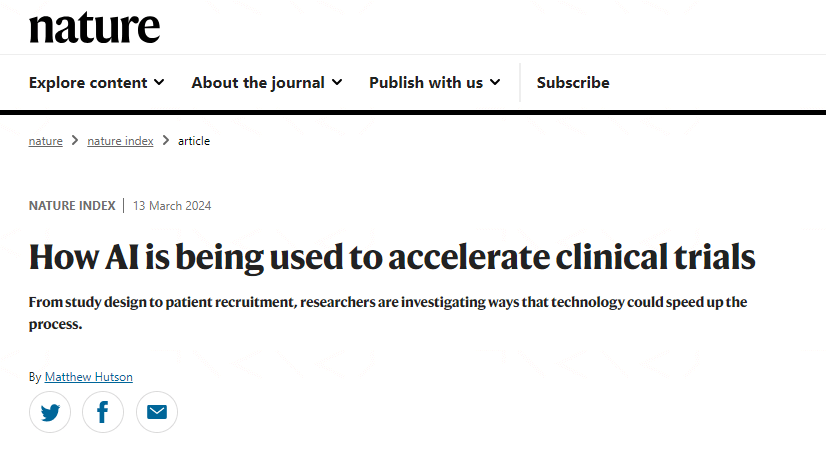
Author
Matthew Hutson
The original article, titled Cutting to the Chase, was published in the Nature Index Health Science supplement of Nature on March 14, 2024. This article adapted and summarized the article without altering the original meaning.
Nature | doi:10.1038/d41586-024-00753-x
Introduction
While Moore’s Law predicted rapid advances in the semiconductor industry, “Eroom’s Law” (Moore spelled backward) illustrates the reverse trend in drug development, highlighting escalating costs and declining efficiency. Over the past 60 years, the number of drugs approved per billion dollars spent on R&D in the U.S. has halved every nine years. Bringing a new drug to market now often exceeds $1 billion in cost and takes nearly a decade, with clinical trials—becoming increasingly larger and complex—accounting for half of that time and expense. Only one in seven drugs entering phase I trials is ultimately approved.
Some researchers hope Moore’s Law innovations can counter Eroom’s Law’s impact. AI has already been employed in early drug discovery to identify disease targets and design molecules. Now, scientists are turning to AI to streamline clinical trials, from protocol design to patient recruitment and data analysis.
Trial Design
The initial step in clinical trials is designing the study. What dosage should be administered? How many patients are needed? What data should be collected? Researchers have developed an algorithm called HINT (Hierarchical Interaction Network) to predict trial success based on drug molecules, target diseases, and patient criteria. They’ve also created SPOT (Sequential Prediction of Trial Outcomes) to aid this process. Additionally, several companies are using OpenAI’s GPT-4 to extract safety and efficacy data from clinical trial summaries.
Patient Recruitment
Patient recruitment is one of the most time-intensive aspects of clinical trials, often consuming up to one-third of the study duration. One in five trials fails to enroll enough participants, and almost all exceed expected recruitment timelines. AI-powered recruitment systems can analyze completed trials and assess how adjustments to inclusion/exclusion criteria might accelerate enrollment.
AI can also streamline redundant tasks, such as optimizing recruitment criteria and automating patient screening. Furthermore, AI-driven systems can match patients to relevant trials more efficiently, enabling researchers and patients to connect faster.
Additionally, digital twin technology could potentially reduce the number of patients required by allowing researchers to predict patient progression in control groups, facilitating result comparisons.
Patient Retention
Patient follow-up is another significant challenge in clinical trials. Analysis of 95 trials reveals that 40% of patients discontinue prescribed medications within the first year. AI-powered chatbots can enhance the patient experience by providing quick, accurate responses to questions and continually improving their answers.
Integrated Functionality
AI also assists researchers in managing and uncovering valuable insights within clinical data, such as extracting information from unstructured reports, organizing text and images, and retrieving billing codes from medical records. This technology can also pull critical data from reports on efficacy, symptoms, side effects, and adverse events.
To collect trial data, researchers sometimes need to generate over 50 case report forms. A Chinese company, Taimei Technology, is leveraging AI to automate this data generation based on trial protocols.
Some companies are working to develop platforms that integrate various AI techniques into a single system. For example, they are using large language models to extract requirements from clinical trial protocols and convert them into structured data. This data can then support other intelligent functions like identifying optimal trial sites, refining inclusion/exclusion criteria, and predicting trial outcomes.
AI is also aiding pharmaceutical companies in preparing clinical trial reports. Intelligent tools can extract relevant data from historical literature or gather insights from social media on diseases and medications, with the information submitted to agencies like the FDA.
Experts suggest that AI will soon be able to handle complex clinical trial design questions, write code to analyze data, and generate visualizations. With the rise of large language models, the potential for this technology is expanding rapidly.
In a recent COVID-19 vaccine trial, AI processed and cleaned data from over 30,000 patients in less than two days—a task that would have taken two months manually.
Challenges
However, experts highlight ethical and practical challenges in deploying AI for clinical trials. AI models can be biased, results may be hard to reproduce, and the algorithms require substantial training data, which raises privacy and security risks. Additionally, reliance on AI could lead researchers to overly depend on technology, while complex algorithms can be difficult to interpret.
“Lack of transparency in clinical trials can be problematic because understanding decision-making processes is essential for trust,” an expert commented. A recent International Journal of Surgery review notes that AI lacks human intuition, common sense, and medical experience.
While transformation in clinical trials is slow, the FDA has relaxed some regulations, and decentralized and remote trials have risen due to the COVID-19 pandemic. Generative AI continues to advance rapidly, though the field has only scratched the surface.
References
Scannell, J. W., Blanckley, A., Boldon, H. & Warrington, B. Nature Rev. Drug. Discov. 11, 191–200 (2012).
Liu, R. et al. Nature 592, 629–633 (2021).
Blaschke, T. F., Osterberg, L., Vrijens, B. & Urquhart, J. Annu. Rev. Pharmacol. Toxicol. 52, 275–301 (2012).
Ayers, J. W. et al. JAMA Intern. Med. 183, 589–596 (2023).
Li, Y. et al. Cureus 15, e40895 (2023).
Chopra, H. et al. Int. J. Surg. 109, 4211–4220 (2023).